The Faculty
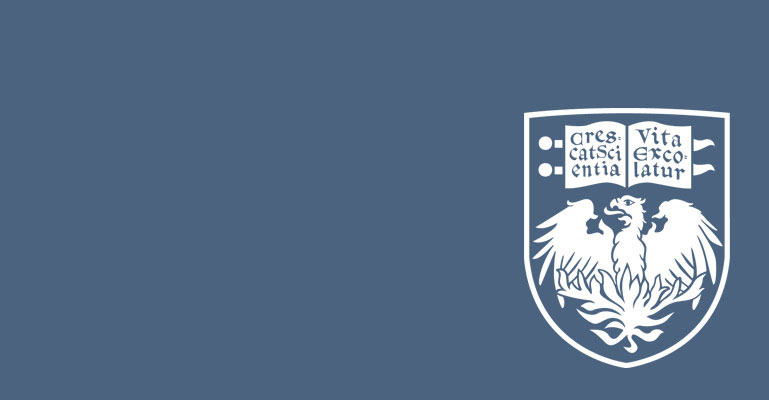
Faculty Photo Coming Soon.
Mengjie Chen
Asst Professor
Affiliation: Department of Medicine (Genetic Medicine)
Websitehttp://www.mengjiechen.com/
Focus
Computational and statistical single cell genomics, transcriptomics and cancer genomics
Research Interests
Single cell genomics
Most of our work focuses on accounting for unwanted variation due to technical artifacts from single cell experiments. Completed and ongoing projects include developing methods to:
control for confounding effects for accurate downstream analysis
preserve gene expression variability across cells after accounting for drop-out and imputing
align single-cell RNA-seq samples across experiments enabling integrative and comparative analyses.
Epitranscriptomics
The paradigm-shifting discoveries in RNA modifications have added RNA to the picture of dynamic regulation of gene expression through reversible chemical modifications. Successful transcriptome wide profiling of RNA modifications can significantly advance the frontier of epitranscriptomics research and associate epitranscriptomic variations with human health and diseases. We are developing methods for the following topics:
Differential methylation analysis
Integrative analysis of m6A modifications with ENCODE/Roadmap data.
Cancer genomics
The cancer genome is characterized by genetic heterogeneity that is seen across tumor types, among samples of a particular type (inter-tumor heterogeneity) and within an individual tumor (intratumor heterogeneity). Understanding tumor heterogeneity is a prerequisite for personalized tumor diagnosis and treatment. We are developing methods for integrative tumor heterogeneity analysis using both single cell RNA sequencing and bulk tissue DNA sequencing data.
Selected publications
Demystifying "drop-outs" in single cell UMI data.
Kim T, Zhou X and Chen M. Genome Biology. 2020 August 06;21(196).
Integrative differential expression and gene set enrichment analysis using summary statistics for scRNA-Seq studies.
Ma Y, Sun S, Shang X, Keller E, Chen M and Zhou X. Nature Communications. 2020 March 27; 11(1585).
Alignment of single-cell RNA-seq samples without over-correction using density matching.
Chen M, Zhan Q, Mu Z, Wang L, Zheng Z, Miao J, Zhu P and Li IY. Genome Research. 2021 March 19. [bioRxiv]
REPIC: A database for exploring N6-methyladenosine methylome.
Liu S, He C and Chen M. Genome Biology. 2020 April 28;21(1):100.
RADAR: Differential analysis of MeRIP-seq with a random effect model.
Zhang Z, Eckret MA, Zhu A, Chryplewicz A, De Jesus DF, Ren D, Kulkarni R, Lengyel E, He C, Chen M. Genome Biology. 2019, 20 (294).
m6A mRNA methylation regulates human β-cell biology in physiological states and in type 2 diabetes.
De Jesus DF, Zhang Z, Kahraman S, Brown NK, Chen M, Hu J, Gupta MK, He C, Kulkarni RN. Nature Metabolism. 2019 Aug;1(8):765-74.
VIPER: variability-preserving imputation for accurate gene expression recovery in single-cell RNA sequencing studies.
Chen M , Zhou X: Genome Biology 2018, 19(1), 196.
The association between copy number aberration, DNA methylation and gene expression in tumor samples.
Sun W, Bunn P, Jin C, Little P, Zhabotynsky V, Perou CM, Hayes DN, Chen M, Lin DY: Nucleic Acids Research 2018, 46(6), 3009-3018.
Integrated RNA and DNA sequencing reveals early drivers of metastatic breast cancer.
Siegel MB, He X, Hoadley KA, Hoyle A, Pearce JB, Garrett AL, Kumar S, Moylan VJ, Brady CM, Van Swearingen AE, Marron D, Gupta GP, Thorne LB, Kieran N, Livasy C, Mardis ER, Parker JS, Chen M, Anders CK, Carey LA, Perou CM: The Journal of Clinical Investigation 2018, 128(4).